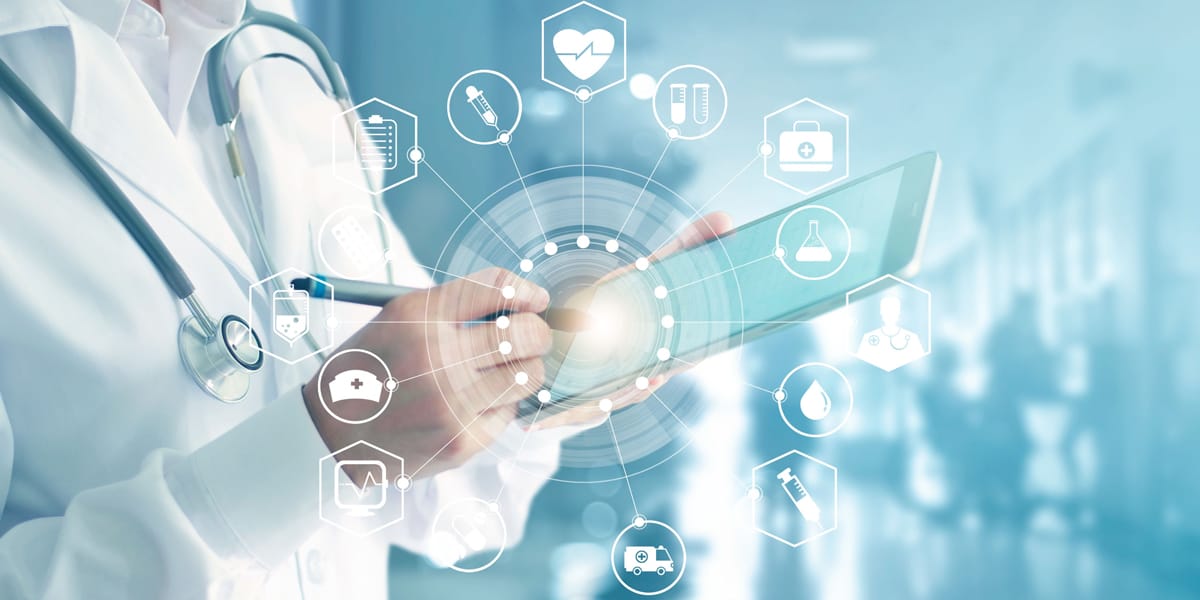
Patient retention is serious business for clinical trials. It’s a complex, time-consuming and costly process to recruit patients, and too many drop-outs can doom a study, prolonging the length of time a product takes to get to market – or preventing it completely.
Not meeting patient recruitment and retention targets is one of the major causes of clinical trial failure. One study of 114 trials in the UK found that only 31% had met their enrollment goals. Furthermore, recruitment and retention issues are responsible for the majority of delays in clinical trial timelines, leading to more costs and a detrimental impact on other areas of the process.
So, what if you could predict that a patient was at risk of leaving a study long before they actually did? What if you could act on that knowledge to address their issues and help them remain in the study?
You would not only save a huge amount of time and money, you would also increase the chances of an important drug reaching the market.
How Can You Predict Patient Sentiment?
Sentiment is not always easy to quantify. Feelings don’t exist in isolated states, and sometimes even a patient can be unclear on their motivations and expectations. Predicting patient sentiment may sound like something from science fiction, but advancements in clinical technology are allowing study organisers to do exactly this with ever-increasing accuracy.
Here’s how we can harness new methods at the forefront of the intersection of technology and linguistics to better understand your patients in exciting new ways.
Humans Talking To Computers
Natural Language Processing (NLP) combines linguistics, semantic tagging, and computer science to investigate the interaction between human and computer languages. One of the greatest benefits of advancements in this field is that computers can be programmed to process and analyse large amounts of textual, transcribed voice, and voice data.
NLP detects the linguistic patterns in text and speech. When combined with machine learning – a branch of artificial intelligence that teaches a computer program to recognise patterns identified via NLP in order to make informed decisions or predictions – this textual/voice data can be used to build an algorithm that can anticipate the future.
For pharmaceutical companies, this means being able to analyse both written and transcribed voice input from patients and use that to make predictions about their future behaviours.
Patient Sentiment Predictions In Practice
There are two main approaches that can be taken to processing textual data: semantic tagging and linguistic parsing.
Semantic Tagging
Semantic tagging means looking for patterns and frequencies in the usage of certain words. This can be useful for identifying important trends that could impact the trial. For example, if a high number of patients report that they “dislike” the Clinical Research Associate, this will be flagged by the system through visual representation (for instance, the word “dislike” could appear larger than any other words reported). This is vital information that can be passed onto the sponsor or CRO to allow them to take action.
Linguistic Parsing
Linguistic parsing is more complex than semantic tagging, as it involves detecting sentiment within a context. Rather than simply looking at raw data with no contextual information, researchers are able to identify and track expressions of specific feelings and understand the influential factors. This is where machine learning can be used to predict how sentiment will develop, and the resulting behaviour – for example, whether a patient will leave a clinical trial.
Using this method therefore not only allows us to identify at-risk patients, but also to recognise what factors are leading them to consider leaving the trial so intervention is possible before they reach that decision. For example, discovering a patient is growing unhappy with travelling four hours to a trial site and may not be willing to continue with this journey could allow sponsors to move to more at-home nursing visits instead, enabling the patient to continue with the trial and be happier about their participation.
The Positive Impact Of Responding To Patient Sentiment
Listening to the sentiment of patients and acting to meet their needs before it’s too late can have a powerful, positive impact on the outcome of clinical trials.
Patient drop-out isn’t the only problem that can be countered by taking patient sentiment seriously. Ensuring patients remain compliant throughout a study is also a major challenge – it’s estimated that 40% of patients become non-compliant 150 days into a trial, for example, missing appointments.
Monitoring and predicting patient sentiment can also identify where patients are likely to lose motivation and stop following the agreed protocol, or where they might be confused, and allow the investigative team to work more effectively and compassionately with them to ensure adherence.
The Rewards Of Accurate Patient Sentiment Prediction
Cost-saving
The average cost of a clinical study is estimated at $42,000 per patient, so repeatedly replacing patients comes with a hefty price tag. One study of cancer trials between 2005 and 2011 found a 20% failure rate, with 48,000 patients enrolled in failed trials. Assuming the average patient cost of $42,000, that is a loss of more than $2 billion.
Data Integrity
Patient drop-outs also negatively affect the study data, potentially undermining the integrity of the trial or – at worst – rendering the statistical data entirely unusable.
Team Morale
80% of clinical trial delays are caused by recruitment and retention issues and on average only one in ten drugs that enter clinical trials making it to market. This puts a huge strain on time and resources, adding additional burden and stress to teams and impacting staff morale.
Accurately understanding, predicting and responding to patient sentiment, therefore, can have huge value. Not only in monetary terms, but for the trial as a whole, the team and the patients. In terms of the bigger picture, if patients participating in a clinical trial feel that their needs are being listened to, considered, and acted upon, they’ll be likely to participate in clinical trials in future, helping more people and getting life-changing products to market faster.
Key Considerations For Implementation
Obtaining Consent
In order to effectively use sentiment prediction and NLP in clinical trials, it is vital that you obtain thorough Informed Consent for the collection, storage and usage of all linguistic data. This will need to be part of the enrollment procedure, along with Institutional Review Board (IRB) ethics approval, and must be incorporated into the informed consent form.
Clear Processes
There will need to be compliant and clear processes in place for obtaining regular patient feedback, through written and/or conversational input. Structures will need to be put in place to ensure the data collected can be effectively recorded and analysed.
The Future Of Sentiment Prediction
NLP and sentiment prediction is still fairly new to clinical trials, and companies are only just beginning to discover the potential of their application for increased patient retention. Over the coming years, these processes are likely to become far more prevalent, and advances in technology will mean predictions become more accurate.
All stakeholders in a clinical trial want a successful outcome, nobody wants the trial to fail. The organisations involved have financial and business motivations, whereas patients simply want to get better. The important thing is for all parties to work together to ensure the trial succeeds.
Patient sentiment prediction is part of a far greater shift towards patient-centricity in all areas of clinical trials. Research shows that drugs developed using a patient-centric approach are 19% more likely to be launched than those that are not. Putting patient sentiment at the forefront gives your study the best chance of success.