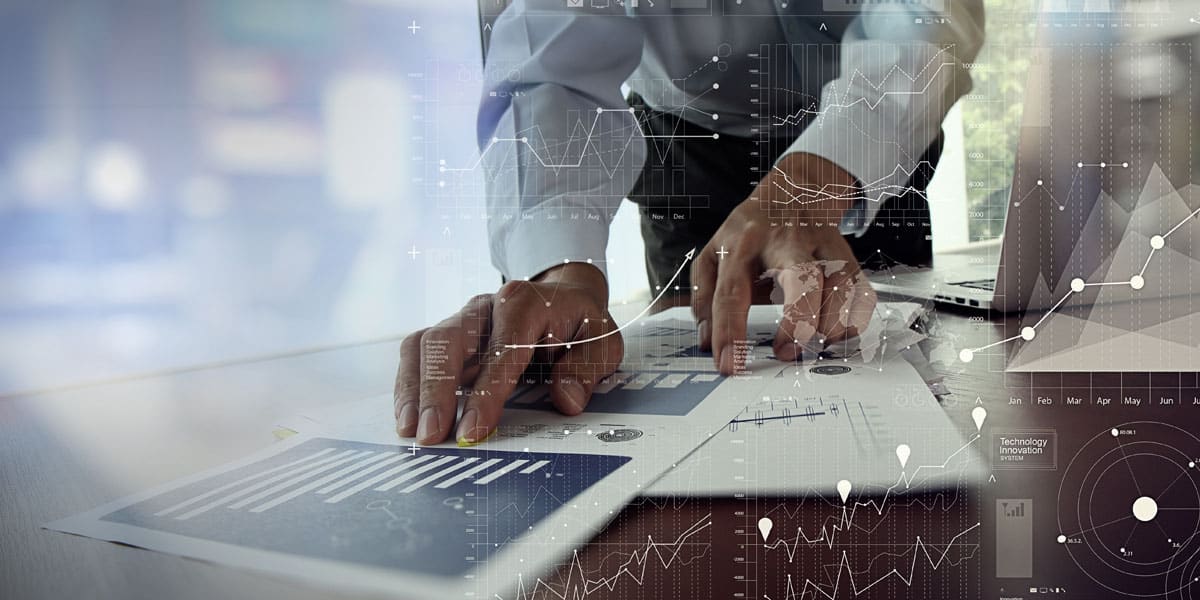
Data capture and analysis is vital in every area of the clinical industry. Increasingly, we’re also seeing that gathering and interpreting data around patient satisfaction and behaviour has a direct impact on patient retention during clinical trials.
By listening to patients’ needs, and collecting data around patient sentiment, CROs, trial designers, and sponsors can identify and fix potential problems, before they’ve even occurred – thus preventing patients dropping out of the clinical trial. Not only does this benefit the integrity of the study and the physical and mental wellbeing of patients taking part, but it also has the potential to save billions in lost revenue, and get products to market faster.
In this article, we look at how data capture, analysis, and reporting can be used effectively to put patients firmly at the centre of clinical trials.
Using Data To Improve Patient-Centricity
Data can come from a variety of sources during a clinical trial. For example, a wearable heart rate monitor can send streams of data to a connected device, informing a healthcare professional of abnormal changes in a person’s heart rate. Patient sentiment can also be captured and monitored over time through a mobile app, allowing investigators to monitor how a patient is doing both emotionally and physically at each stage of the clinical journey.
Effective and reliable capture is the first step in the process of harnessing data to improve the patient experience, whether you are monitoring a person’s health or listening to their needs.
To give a tangible example, our mobile app, primarius is designed to proactively inquire about how a patient feels about the clinical trial they’re participating in. By capturing data around patient sentiment in this structured, quantifiable way, we’re better able to anticipate and fix any issues that may lead to the patient dropping out of the study.
Data in this context is only useful when it becomes actionable. If the data informs you that a patient is thinking of leaving the trial (for example, if they indicate that they are finding travel difficult, or that they are dissatisfied with the level of care they are receiving), you should be able to take action to remedy the issue. However, for the data to become actionable, it needs to be structured and measurable so that it can be analysed and interpreted correctly.
Data analysis is the “hard part” of data processing. This is where data specialists identify, categorise, and measure the information gathered, looking for predictive patterns and correlations. A good data analysis will be able to identify and communicate the story behind the data, which is when data becomes useful.
With effective reporting, findings from the data analysis are communicated to all relevant stakeholders in a way that is relevant and applicable to the study. Reports should make it clear when and how sponsors or CROs should and can intervene before a patient decides to leave the clinical trial.
Common Pitfalls In Processing Clinical Trial Data
Data captured during a clinical trial can come from a variety of sources, including multiple processes, systems or devices. Usually, data capture occurs automatically in real-time through an Application Programming Interface. This means that all of this unstructured data comes at once, like a tsunami wave that we need to structure and make sense of.
Due to their complexity, clinical data sources can be riddled with errors, which is a major problem for clinical trials. Electronic health records (EHR) are notorious for this, as are real-world data (RWD) sources.
Patient data captured during clinical trials is also liable to bias, as patients will often provide the answers they think investigators want to hear. To counteract this effect, trial designers should ask the same questions in a variety of ways, and capture data in a real-world setting wherever possible to avoid phenomena such as white coat syndrome.
Finally, data gaps may occur when patients do not communicate as generously or record as rigorously as they should. These missing or incomplete data sets can lead to an incorrect analysis.
Overcoming Common Data Pitfalls
It’s crucial for us that our clients receive “clean” data – that is, data that is well structured and free from errors. To ensure this, we use a Master Data Management (MDM) strategy which makes it possible for clients to link all critical data to a common point of reference. Master Data Management improves the quality of the data, as it allows data to be arranged and interpreted in a systematic way while also “cleansing” it when it enters the system.
To give an example of this in action, as part of our patientprimary service, we capture all transactional data regarding travel, accommodation, and out-of-pocket reimbursement costs in one location. Then, we compare these costs against a common point of reference: our client’s budget.
Since costs can fluctuate due to numerous factors, our primarius app can flag a cost excursion that is above the client’s budget threshold before it becomes critical. Data analysis is therefore used to anticipate and flag potential issues, rather than the client having to interrogate the system only to discover that there are budgeting errors or that the patient is in trouble.
In terms of data around patient sentiment, technology can record and analyse how a patient feels physically as well as emotionally about the clinical trial at regular intervals throughout the entire process.
Capturing patient sentiment in an organised, structured way is crucial because then this becomes actionable data. We’re then able to structure and analyse the data using machine learning, informing our clients about which patients are leaning towards dropping out so that this can be rectified.
Six Ways Data Can Be Used To Improve Clinical Trial Outcomes
Data is crucial to the success of a clinical trial. Of course, without data, there can be no validation of a clinical trial’s success. But, as we have discussed, data can do a lot more than this. Data capture, analysis, and reporting can enhance patient retention by making trials more patient-centric in numerous ways.
1. Keeping patients safe
A variety of data sources (whether transactional, physiological, or linguistic) provide the information needed to determine positive and negative changes to a patient’s health and wellbeing. Adverse health effects can therefore be quickly identified before they become dangerous or threaten patients’ wellbeing.
2. Improving patient satisfaction and patient retention
Data provides a vehicle for meaningful two-way communication with patients. It can provide experiential evidence that a drug isn’t working at a certain dosage which signals to HCPs to intervene, or that travel is having a detrimental impact on a patient’s health so that home visits might be considered where possible.
3. Delivering actionable results
Once analysed properly, data becomes actionable. This means it tells study organisers when and how to intervene before circumstances become critical – whether that’s a patient leaving a trial or an adverse health event.
4. Reinforcing focus on the patient
All the ways of using data we have discussed are concerned with supporting patients along the clinical trial journey and making their experience better. With every bit of data analysed and reported on, sponsors are reminded that patient centricity is key for successful clinical trials.
5. Creating a pool of shared knowledge
Patient data can be shared with everyone involved in a clinical study, from the CRO to the sponsors, sites, and regulatory agencies. This creates a pool of shared knowledge, which helps everyone learn from the process and means that future studies can be improved.
6. Increasing accountability
Reporting on data within a shared “ecosystem” of knowledge holds everyone involved in the clinical trial accountable for its success in a transparent, informed manner. Using data to determine clear, measurable actions ensures that everyone is accountable.
In Summary
An increasing number of studies focusing on patient centricity and improving patient retention in clinical trials have stressed the importance of holistic data for creating a safer clinical trial journey with better patient outcomes.
Without a systematic, reliable data capture strategy combined with effective data analysis and actionable reporting, there would be no reportable patient outcomes. Effective data capture, analysis, and reporting across all areas of a clinical trial are therefore essential in protecting patients physical and mental wellbeing, ensuring patient satisfaction, and ensuring patient retention. When data is then used to shape more patient-centric clinical trial designs in the future, this will likely have a positive impact on patient recruitment.